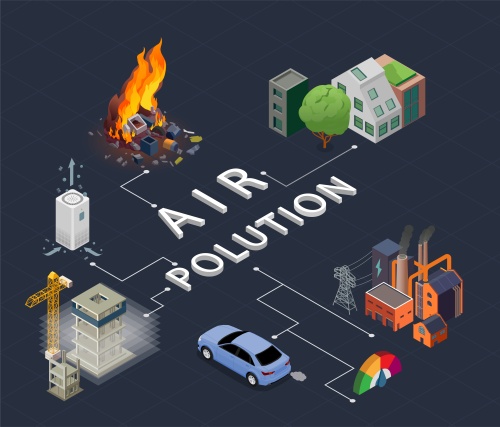
As the world faces an increasing number of natural and man-made disasters, from hurricanes and earthquakes to industrial accidents and pandemics, the role of technology in disaster prevention has never been more critical. Technological advancements have provided new tools for forecasting, mitigating, and even preventing disasters before they occur. These technologies offer the potential to save lives, reduce economic losses, and help communities recover more quickly. However, as with any innovation, there are challenges and risks associated with their use, ranging from data privacy concerns to the reliability of predictive models. This essay explores the significant role technology plays in disaster prevention efforts, focusing on early warning systems, predictive modeling, remote sensing, and the use of artificial intelligence.
Early Warning Systems: Preparing for the Unexpected
One of the most important applications of technology in disaster prevention is the development of early warning systems (EWS). These systems, which use a combination of sensors, satellite data, and communication networks, are designed to provide timely alerts about impending natural disasters, such as hurricanes, tsunamis, earthquakes, and volcanic eruptions. By giving communities advanced notice, early warning systems enable people to evacuate, secure property, and prepare for the worst, reducing the impact of the disaster on lives and infrastructure.
For example, in the case of hurricanes, modern satellite technology and weather models are used to track storm formations, predict their paths, and estimate their intensity. This data is then fed into EWS, which broadcasts warnings through various channels such as television, radio, mobile apps, and social media, ensuring that the information reaches as many people as possible. Similarly, in the event of an earthquake, seismographs and ground sensors can detect tremors and send alerts within seconds, allowing individuals and communities to take cover or prepare for aftershocks.
In some cases, early warning systems also provide specific instructions, such as evacuation routes or shelter locations, tailored to the nature of the disaster. The faster and more accurate these warnings are, the better the chances of saving lives and reducing damage. These systems have become an essential part of disaster management in many countries, particularly those with high disaster risks, such as Japan, Indonesia, and the United States.
Predictive Modeling: Anticipating Disaster Risks
Another critical area where technology plays a role in disaster prevention is predictive modeling. Predictive models use historical data, real-time information, and advanced algorithms to forecast the likelihood of disasters occurring in specific regions. These models are invaluable for disaster planning, as they help governments, organizations, and communities assess risks and allocate resources more effectively.
For instance, predictive modeling in flood prevention uses rainfall data, topography, and historical flood records to estimate areas at risk of flooding. This allows authorities to implement flood management strategies, such as constructing dams, flood barriers, or improving drainage systems, in advance of heavy rains. Similarly, predictive models for wildfires analyze weather conditions, vegetation types, and historical fire data to determine which areas are most susceptible to fires, enabling targeted fire prevention and mitigation measures, such as controlled burns and firebreaks.
Predictive models are also widely used in public health and pandemic management. During the COVID-19 pandemic, data-driven models were used to predict the spread of the virus, assess the impact of different interventions, and inform public health policies. By simulating various scenarios, these models provided valuable insights into how diseases might spread, helping governments and healthcare systems prepare and respond more effectively.
While predictive modeling has proven effective in many contexts, it is not without its limitations. The accuracy of predictions depends on the quality and availability of data, and unforeseen factors—such as human behavior or environmental changes—can lead to deviations from predictions. However, advancements in machine learning and AI are improving the accuracy and adaptability of predictive models, making them more reliable over time.
Remote Sensing: Monitoring the Environment in Real-Time
Remote sensing technology, which involves collecting data from satellites, drones, and ground-based sensors, plays a vital role in monitoring the environment and detecting potential hazards. These technologies are essential for tracking changes in the environment that could lead to natural disasters, such as shifts in weather patterns, soil erosion, or the weakening of infrastructure.
For example, satellite imagery can be used to monitor deforestation, which increases the risk of landslides and floods, particularly in mountainous regions. By detecting changes in vegetation or soil moisture, remote sensing can help identify areas at risk and trigger early warnings for communities downstream. In coastal regions, remote sensing is used to monitor rising sea levels, enabling authorities to assess the risks posed by storm surges and flooding.
Drones, too, have proven to be effective tools for disaster prevention. Equipped with high-resolution cameras and sensors, drones can survey areas that are difficult to access, such as remote forests or disaster-prone urban areas. Drones can provide real-time data that is used for assessing risks, monitoring hazards, and inspecting infrastructure. After a disaster, drones are often used to assess damage, locate survivors, and assist in recovery efforts.
Artificial Intelligence: Enhancing Decision-Making and Automation
Artificial intelligence (AI) is playing an increasingly significant role in disaster prevention, particularly in enhancing decision-making and automating key processes. AI-powered systems can analyze large volumes of data, identify patterns, and provide insights that improve disaster preparedness and response.
One area where AI is making a difference is in predictive analytics. AI can process vast amounts of environmental data, such as weather patterns, seismic activity, and ocean currents, to predict the likelihood of disasters such as storms, earthquakes, or tsunamis. These predictions can be combined with early warning systems to provide faster, more accurate alerts, enabling better preparedness and quicker response times.
AI is also being used to improve disaster response coordination. In the aftermath of a disaster, AI-driven systems can analyze social media posts, emergency calls, and sensor data to identify areas that require immediate assistance. This helps optimize resource allocation, ensuring that help reaches the most affected areas quickly.
Additionally, AI is being integrated into the design and construction of disaster-resistant infrastructure. Machine learning algorithms can be used to simulate how buildings, bridges, and roads will perform in the event of a disaster, helping engineers design structures that are more resilient to earthquakes, floods, and other hazards.
Challenges and Limitations
While technology has proven to be invaluable in disaster prevention, there are several challenges that need to be addressed. One of the primary concerns is the accessibility and availability of technology in disaster-prone regions. Many developing countries and remote areas lack the infrastructure and resources to implement advanced technology, which can hinder the effectiveness of disaster prevention efforts.
Moreover, the reliance on technology raises concerns about data privacy and security. With the increasing use of sensors, drones, and satellite imagery, vast amounts of data are being collected, often involving personal information or sensitive environmental data. Ensuring that this data is protected from unauthorized access or misuse is crucial for maintaining public trust and ensuring the long-term success of technology-driven disaster prevention initiatives.
Conclusion
Technology has revolutionized disaster prevention efforts, offering innovative solutions for forecasting, mitigating, and managing disasters. From early warning systems and predictive modeling to remote sensing and artificial intelligence, these technologies have significantly improved our ability to prevent, respond to, and recover from disasters. However, challenges remain in terms of accessibility, data security, and the need for continuous innovation. By addressing these challenges and ensuring that technology is used responsibly and inclusively, we can further enhance our capacity to prevent disasters and protect vulnerable communities. With ongoing advancements in technology, the future of disaster prevention holds great promise, offering hope for a more resilient and prepared world.